Orchestrate AI workloads in any cloud
dstack is an open-source orchestration engine for running AI workloads at scale in any cloud or data center, leveraging the open-source ecosystem.
Dev environments
Before scheduling a task or deploying a model, you may want to run code interactively.
Dev environments allow you to provision a remote machine set up with your code and favorite IDE with just one command.


Tasks
Tasks allow for convenient scheduling of various batch jobs, such as training, fine-tuning, or data processing, as well as running web applications.
You can run tasks on a single machine or on clusters.
Services
Services make it very easy to deploy any kind of model as public, secure, and scalable endpoints.
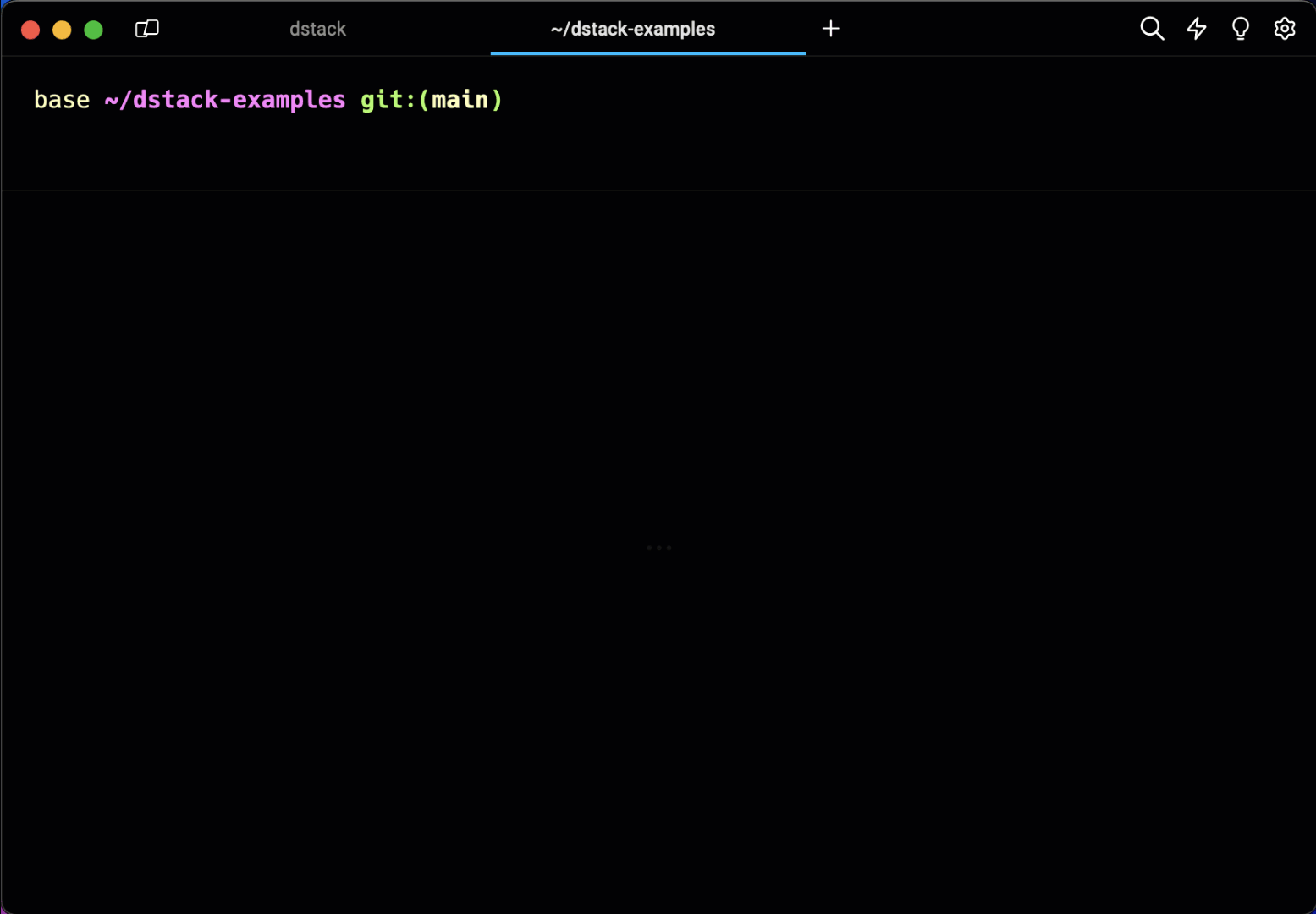
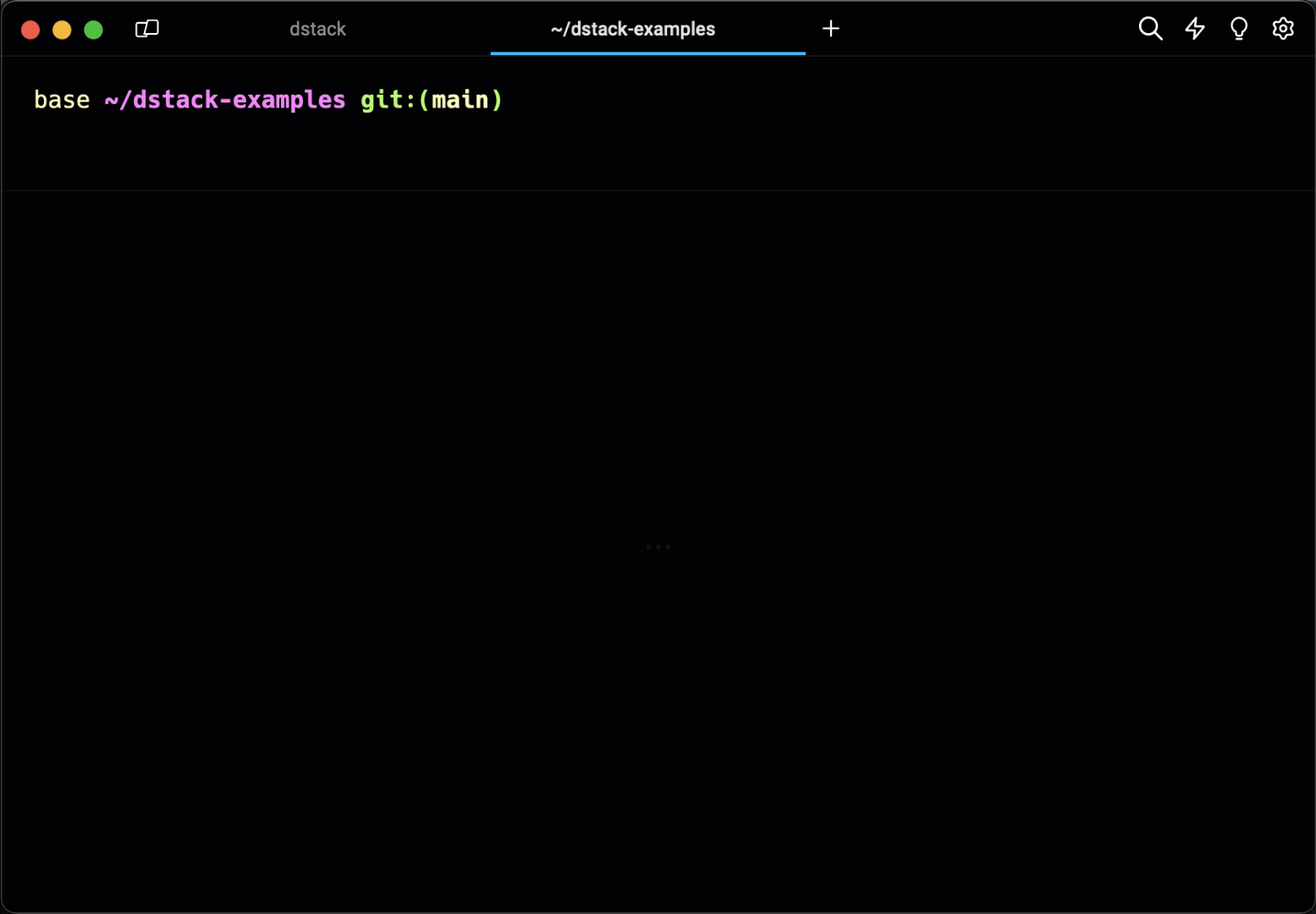
Pools
You can have instances provisioned in the cloud automatically, or add them manually, configuring the required resources, idle duration, etc.
Pools simplify managing the lifecycle of cloud instances and enable their efficient reuse across runs.
Examples
Llama 3
Deploy Llama 3 as a service using Ollama, an open-source serving tool.
QLoRA
Fine-tune Llama 2 on a custom dataset using the PEFT and TRL, open-source training libraries.
vLLM
Deploy LLMs with vLLM, an open-source serving library.
Text Generation Inference
Deploy LLMs using TGI, an open-source serving library.
Ollama
Deploy LLMs using Ollama, an open-source serving tool.
Text Embeddings Inference
Deploy a text embeddings model using TEI, an open-source serving library.